Listening to the Wild: How NatureLM Is Decoding Animal Communication Through AI
- hashtagworld
- Apr 10
- 3 min read
Earth Species Project's open-source AI model marks a paradigm shift in our relationship with non-human intelligence.
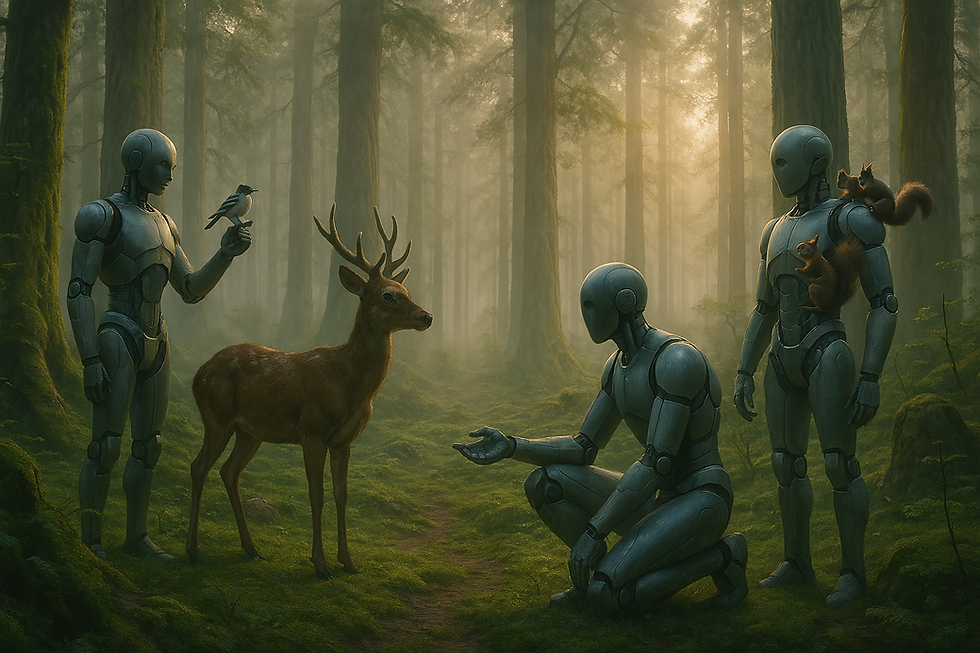
Introduction
Artificial intelligence (AI) has long been confined to human languages refining search engines, generating art, and drafting essays. But a quiet revolution is emerging at the edge of bioacoustics and machine learning: AI models that listen not to humans, but to the wild.
At the forefront of this movement is NatureLM, a large language model trained not on text, but on the soundscapes of animal life. Developed by the nonprofit Earth Species Project (ESP), NatureLM aims to decode animal communication using AI techniques inspired by the human-centric GPT architecture. Its implications reach far beyond biology toward a future where communication across species is not a fantasy, but a functional scientific endeavor.
1. What Is NatureLM and Who’s Behind It?
Founded in 2017, Earth Species Project is an interdisciplinary research initiative that applies machine learning to non-human communication. Collaborating with bioacousticians, conservationists, and AI researchers, ESP’s goal is to open-source technologies that accelerate understanding between humans and other species.
NatureLM is ESP’s flagship project. Inspired by transformer-based language models like GPT-3 and BERT, it applies similar deep learning frameworks to acoustic datasets of whales, primates, birds, elephants, and more. Instead of predicting the next word in a sentence, NatureLM predicts, classifies, and clusters vocal patterns based on context and meaning without needing direct translations.
2. How NatureLM Works
NatureLM leverages a self-supervised learning architecture. Just as large language models learn from billions of words in context, NatureLM learns from sequences of sound such as whale clicks or bird calls to identify recurring structures and potential semantic relationships.
Key methodologies include:
Contrastive Learning: Differentiating between similar and dissimilar sound segments.
Clustering Algorithms: Identifying categories of vocalizations with shared acoustic features.
Representation Learning: Embedding animal vocalizations into high-dimensional space for pattern analysis.
One of the most innovative aspects of NatureLM is its cross-species transferability: its representations can generalize across species, revealing previously unknown parallels in communication strategies.
3. Scientific Implications
NatureLM could fundamentally reshape fields such as:
Animal Cognition: Helping researchers understand how animals encode intention, emotion, or spatial information in sound.
Conservation Biology: Identifying distress calls or ecosystem disruption signals in real time.
Taxonomy: Revealing vocal signatures unique to populations or even cryptic species.
Behavioral Ecology: Mapping complex social dynamics through acoustic interactions.
With NatureLM, data from bioacoustic monitoring stations once ignored due to the sheer volume becomes fertile ground for discovery.
4. Ethical and Philosophical Dimensions
Decoding animal communication is not without controversy. As with all AI systems trained on language, interpretation bias is inevitable. Are we truly understanding animal intent, or projecting human categories onto their sounds?
This raises deeper questions:
Can animals consent to being studied with such invasive precision?
Should decoded animal messages influence policy or legal frameworks?
Are we witnessing a new form of digital colonization this time of non-human minds?
ESP addresses these concerns by grounding its research in open science and transparency, acknowledging that interpretation must always be cautious and collaborative.
5. Potential Applications
Though still early in development, NatureLM could have far-reaching applications:
Wildlife Conservation: Detecting illegal poaching activity through unusual animal vocal patterns.
Veterinary Science: Early detection of illness or stress in domestic or captive animals.
Climate Monitoring: Using shifts in natural soundscapes to infer ecological changes.
Education & Awareness: Helping humans "hear" nature in new ways, deepening empathy for other species.
As AI continues to evolve, its capacity to serve as an interpreter between lifeforms opens a new philosophical dimension of technology.
6. Rethinking the Role of AI
NatureLM challenges the mainstream narrative of AI as a productivity tool. Instead, it repositions AI as a mediator, one that can bridge the epistemic gap between species that have coexisted for millennia but never understood each other.
In doing so, AI becomes more than machine intelligence it becomes ecological intelligence, embedded in the rhythms of life itself.
Conclusion
NatureLM is not just an algorithmic breakthrough; it’s a conceptual one. It invites us to question not only how AI can "understand" animals, but also how we define communication, consciousness, and kinship in the natural world.
In a time when biodiversity is under threat and interspecies empathy is desperately needed, NatureLM represents a hopeful path forward. It does not give voice to the voiceless rather, it helps us finally listen.
References
Earth Species Project – Official Website🔗 https://www.earthspecies.org
NatureLM Model Release – Earth Species Medium Post🔗 https://medium.com/earthspecies/naturelm-open-sourcing-a-language-model-for-the-natural-world-6eb79b8384d9
WIRED – Can AI Help Us Talk to Whales?🔗 https://www.wired.com/story/earth-species-project-ai-animal-communication
Stanford HAI – Non-Human Communication Research🔗 https://hai.stanford.edu/news/ai-translating-animal-communication-possible
arXiv – Machine Learning for Bioacoustics🔗 https://arxiv.org/abs/2209.13198
コメント