Securing the Mind of the Machine: MIT’s Breakthrough in Ethical AI Training
- hashtagworld
- Apr 11
- 3 min read
Can we teach AI without compromising our data or our values?
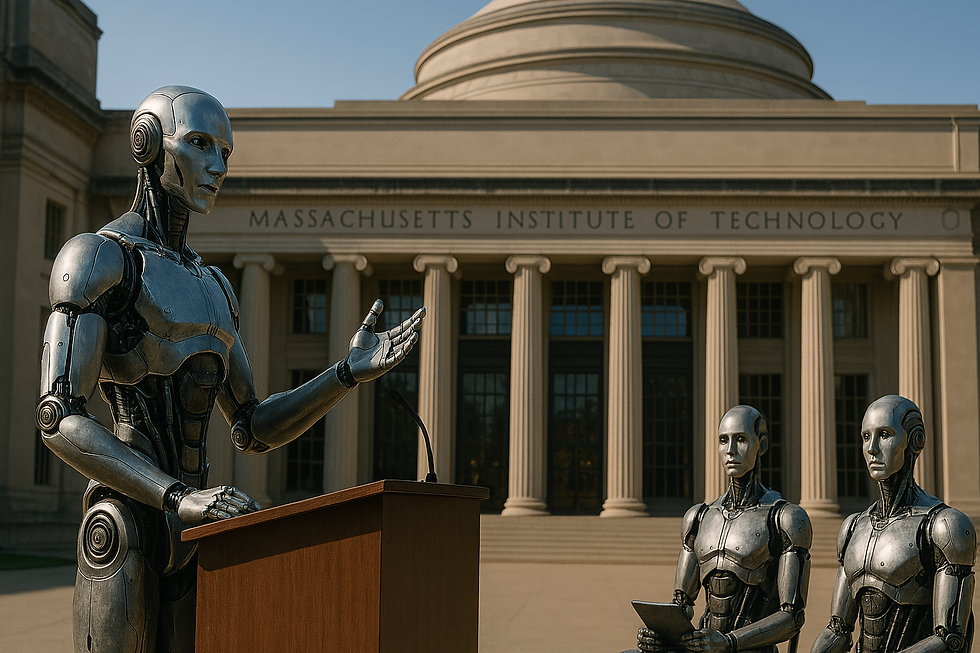
Introduction
As artificial intelligence systems grow more powerful, so do the ethical challenges surrounding their development. Chief among them is the tension between performance and privacy. Training AI requires vast datasets often containing sensitive, personal, or demographically skewed information. But how can we harness this data responsibly without sacrificing accuracy or fairness?
MIT researchers have recently introduced a groundbreaking technique aimed at resolving this paradox. Their method allows developers to preserve model performance while minimizing bias, overfitting, and exposure of sensitive training data all without altering the AI model’s internal architecture. This breakthrough may become foundational in building a new generation of trustworthy, transparent, and human-centered AI systems.
1. The Data Dilemma in AI Development
Modern AI models are data-hungry. Whether it's medical imaging, hiring algorithms, or customer profiling tools, these models often learn from historical datasets that are far from neutral. The risks are twofold:
Privacy Leaks: Language models trained on unfiltered internet data may inadvertently memorize and reproduce personal information.
Structural Bias: If historical data reflects societal discrimination, the AI is likely to replicate—and even reinforce those patterns.
Until now, mitigating these issues typically involved removing entire demographic subsets or retraining models from scratch both costly and imperfect solutions.
2. MIT’s Targeted Data Filtering Method
The innovation from MIT proposes a more elegant, surgical approach:
Step 1: Identify MisclassificationsThe model’s incorrect predictions especially toward minority groups are mapped with precision.
Step 2: Trace Responsible DataUsing a method called TRAK (Tracing Attack), researchers determine which specific training samples most contributed to those errors.
Step 3: Prune, Don’t PurgeInstead of scrapping entire segments, only the problematic samples are removed, preserving the bulk of the data and performance.
This is achieved without modifying the model’s internal weights or architecture, which is particularly beneficial for large pre-trained systems like GPT or BERT.
3. Why This Matters for the Future of AI
This method is significant for several reasons:
Data-Efficient FairnessIt allows developers to achieve fairness without trading off massive amounts of valuable data.
Architecture-AgnosticIt can be applied to any model no need for retraining from zero or customizing neural layers.
Security EnhancementSensitive or harmful data points can be targeted and removed even after training critical in large-scale foundation models.
Ethics-by-DesignIt aligns with the principle of building ethical infrastructure into the foundation of AI not retrofitting fixes after deployment.
4. Real-World Use Cases
Imagine a healthcare diagnosis model that consistently underdiagnoses symptoms in Black patients. With MIT’s method, hospital IT teams could pinpoint and remove the specific data samples contributing to that disparity without compromising the model’s accuracy for all patients.
Or consider a content moderation system that flags African-American vernacular English more frequently as toxic. With this technique, the platform could refine its training data to correct the bias without rebuilding the entire moderation engine.
5. Ethical and Social Impact
This method introduces not just a technical upgrade, but an ethical one. It reinforces the idea that:
Data responsibility is not optional.
Bias is not an inevitable artifact.
AI systems can and must be audited, refined, and made accountable.
In an era of accelerated AI deployment, tools like these may prove essential in bridging the trust gap between technology and society.
Conclusion
As artificial intelligence becomes woven into the fabric of modern life, its impact on privacy, fairness, and transparency will only grow. MIT’s approach represents more than a clever engineering fix it is a moral statement, demonstrating that precision and ethics can coexist in machine learning.
The future of AI must be both powerful and principled. And thanks to innovations like these, we’re one step closer to making that future real.
References
MIT News – New method efficiently safeguards sensitive AI training data (April 2025)🔗 https://news.mit.edu/2025/new-method-efficiently-safeguards-sensitive-ai-training-data-0411
CSO Online – OpenAI slammed for putting speed over safety🔗 https://www.csoonline.com/article/3960456/openai-slammed-for-putting-speed-over-safety.html
arXiv – Tracing Training Data in Deep Learning Models (TRAK Method)🔗 https://arxiv.org/abs/2302.12387
The Times – AI forecast to fuel doubling in data centre electricity demand by 2030🔗 https://www.thetimes.co.uk/article/ai-forecast-to-fuel-doubling-in-data-centre-electricity-demand-by-2030-htd2zf7nx
Stanford HAI – Ethics and Responsible Data Use in AI🔗 https://hai.stanford.edu/news/ethics-responsible-data-use-ai
Comments